In 1961, Francois Jacob and Jacques Monod discovered
the biology of gene expression and gene regulation.
Their pioneering work began to explain how a
static DNA genome can adapt and respond to a constantly
changing environment. But it would be
another 28 years until scientists could analyze
expression from more than a few genes at a time.
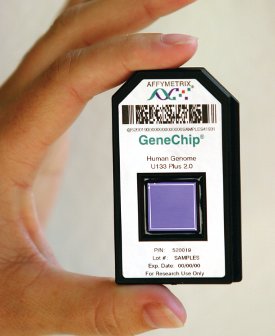
Figure 1 - The GeneChip Human Genome U133 Plus 2.0
array offers researchers the protein-coding content of the human
genome on a single commercially available catalog microarray.
The 1989 invention of the DNA microarray by Stephen
P.A. Fodor and colleagues1–3 provided researchers with the
ability to analyze expression from thousands or even tens
of thousands of different genes simultaneously. Up until
that point, researchers picked two or three genes they
believed were central to a certain disease and spent years
or even entire careers studying just that handful of genes in
the hope of finding some causal link to the disease. Using
GeneChip® arrays (Affymetrix, Santa Clara, CA),
researchers no longer need to hypothesize about which
genes to focus on; instead they can look at the entire
genome at one time and compare disease-state expression
to a normal control (Figure 1). This objective analysis
method enables scientists to discover the underlying
genetics and associated biochemical pathways that are disrupted
in a wide range of diseases, from cancer4–6 to multiple
sclerosis.7 There are literally hundreds of examples, presented
in nearly 3000 scientific publications, in which
GeneChip technology has been used to extend our understanding
of disease and to identify the molecular pathways
that modulate it. Furthermore, the successful use of expression
profiling to classify complex diseases enables
researchers to identify molecular mechanisms that are
more likely to be causative of disease and therefore better
diagnostic indicators or possible therapeutic targets.
The same characteristics that make GeneChip expression
arrays so ideally suited for basic disease research—high data capacity, reproducibility, and accuracy—have
allowed the technology to revolutionize drug discovery
and clinical research and development. High-data-capacity
GeneChip microarrays have been adopted by
pharmaceutical companies for disease-pathway target
validation, compound profiling, and toxicology studies.
Additionally, the arrays are currently being used in
dozens of clinical trials to stratify disease, predict patient
outcome, and generate information that can be used to
make better therapeutic choices. From scientific obscurity
15 years ago, the now widespread use of GeneChip
expression arrays is reviewed, from the laboratory bench
to the industrial and clinical arenas, demonstrating the
highly scaleable nature of GeneChip technology and its
ability to provide more in-depth analysis of the genome.
Data capacity
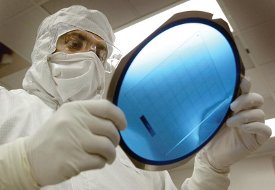
Figure 2 - Multiple GeneChip arrays are simultaneously synthesized
on a single 5-in. wafer. One wafer can be cut into thousands
of separate arrays.
Researchers use GeneChip arrays in all areas of disease
research because of their ability to measure massively
parallel gene expression. GeneChip microarrays are a
classic Silicon Valley innovation, integrating semiconductor
fabrication techniques with random-access
combinatorial chemistry (Figure 2). This results in a
scaleable photolithographic manufacturing process
capable of producing GeneChip arrays with millions of
probes on a single glass chip about the size of a dime.
As in semiconductor fabrication, photolithography has
enabled Affymetrix to increase the amount of information
it can fit on each successive generation of arrays.
Every time the company reduces the size of microarray
features by two, it gains a fourfold increase in data
capacity. The first commercial microarray, produced by
Affymetrix in 1994, accommodated 16,000 probes. Its
most recent human expression array accommodates
over 1.3 million probes, able to measure expression for
all known coding DNA in the human genome (nearly
60,000 transcripts) with 11-fold redundancy.
Industrialized research
Commonly used to identify genes and pathways responsible
for disease, GeneChip expression arrays are well suited
to validate those disease pathways and screen potential
drug compounds for treatment efficacy and toxicity.
Disease pathway target
validation
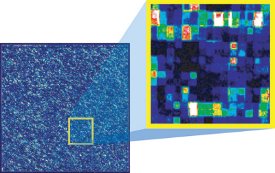
Figure
3 - A computer readout from a scanned microarray
shows the genes that are detected by a single GeneChip probe array.
When scientists zoom in, they can see the different levels of fluorescence
coming from the individual probe locations. Some probes
detect intense gene expression (bright white and red features) and
some do not (dim blue and black features).
Once a disease pathway is identified, researchers need to
know that disrupting the pathway will actually affect the
disease etiology. Using whole-genome expression profiling
(Figure 3), scientists can understand a wide range of effects,
desirable and undesirable, that result from disrupting a
pathway and are then able to better evaluate potential targets
for drug design. Modern technologies, such as small
interfering RNA, are now being used to rapidly and specifically
inhibit gene function, speeding up the exploratory
process of validating useful drug targets. However, being
able to affect many different genes quickly requires an
equally efficient way to measure the downstream effects
generated by those changes.8 Additionally, custom resequencing
microarrays can be used to pinpoint disease-causing
mutations or to measure the genetic variability of
a target gene in clinical populations. The information generated
from GeneChip microarrays gives researchers a
more complete understanding of how a gene functions
within a cell and adds significant value to the biological
models used to validate gene targets.
Compound screening:
Mechanism of action
Following disease pathway identification and validation,
whole-genome microarray analysis can be used to characterize
lead compounds for selectivity and specificity, and to
identify molecules that disrupt expression of intended disease
genes. While existing technologies are well suited to
measuring the anticipated action of a development compound,
these methods do not typically identify any additional
or unexpected effects. Whole-genome expression
analysis provides a complete and unbiased measure of both
on- and off-target effects for each compound tested.
Clearly, on-target effects are desired; however, off-target
changes in expression may help treat different diseases,
operating through a different mechanism. For example,
despite their development to treat hypertension and
depression, the respective blockbuster successes of Viagra®
(Pfizer Pharmaceuticals, New York, NY) for erectile dysfunction
and Wellbutrin® (GlaxoSmithKline, London,
U.K.) for smoking cessation are prime examples of exploiting
off-target drug action to serve other therapeutic markets.
By developing large databases of information on the
global activity of each member of a compound library, microarray expression analysis allows companies to ultimately
create “smarter” compound libraries, with recorded
and known effects for each member compound.
Compound screening:
Mechanisms of toxicity
Microarray gene expression screening not only helps to
identify mechanisms of drug action, but also points to
other off-target effects that may suggest the compound
produces far too many side effects to be approved. For
instance, if changes in gene expression match those of a
known toxin, a compound can be eliminated from the
screening process early in development, saving both time
and money. Compound toxicity is typically not evaluated
until later stages in the development pipeline and has
become a major reason for the high attrition rate in drug
development. In the past, the belief has been that once a
compound is found to be active, it can be sufficiently
modified to avoid toxic effects while retaining its specific
activity.9 However, a recent review of the literature
demonstrates that, generally, successfully developed drugs
undergo few modifications from their initial lead form.10
Using microarrays to understand a compound’s risk profile
earlier in the development process allows for more efficient
and cost-effective decision making regarding compound
prioritization for future drug development.
Clinical research
The benefits of gene expression analysis are not limited
to the initial stages of drug development. GeneChip
expression arrays can be further used in clinical research
and disease classification, areas with direct impact on
human health.
Classifying disease
The first step in treating patients is to correctly identify
their diseases. This has typically been performed by classical
clinical exams, histological data, and laboratory tests.
However, genome-wide expression profiling of diseases
like breast cancer, leukemia, and prostate cancer have
provided for more precise disease classifications and have
revealed that similar tumor types have distinct molecular
differences.11 This explains why clinicians have been baffled
for years when, for instance, two breast tumors looked
identical, but patient response to treatment and patient
outcomes were radically different. In reality, the patients
had different diseases, and different diseases require different
treatments. By more accurately classifying the molecular
nature of the disease, clinicians would be able to
choose and design more effective treatments.
Nowhere is this fundamental change more profound than
in cancer research, where hundreds of microarray studies
are offering new hope for diagnosing, classifying, and treating
both common and rare cancers. Studies on medulloblastoma,12 prostate cancer,13 breast cancer,14,15 lung
cancer,16 colon cancer,17 renal cell carcinoma,18 and diffuse
large B-cell lymphoma19 are just a few examples of
cancers in which established gene expression classification
systems have been developed, often offering important
prognostic indications for cancer outcome and recurrence,
as well as patient response to treatment. Studies have thus
far been retrospective, but future maturation and commercialization
of the technology promises prospective uses in
the clinic and direct impact on patient treatment.
In the realm of initial disease identification, gene expression
patterns may provide early, noninvasive clues to the
detection of deep internal organ malignancies. For example,
as part of a Phase II clinical study studying renal cell
carcinoma,18 Wyeth (Madison, NJ) used GeneChip
expression arrays to profile gene expression from peripheral
blood. They found a specific set of expressed genes
that could be used to distinguish blood cells from renal cell
carcinoma patients and normal volunteers with high accuracy.
Use of peripheral blood gene expression as a diagnostic
marker for disease has important implications for both
the clinical diagnosis and future clinical pharmacogenomic
studies of antitumor therapies.
Predicting drug response
The efficacy of even the most successful drugs can vary
widely from individual to individual. Whole-genome
expression arrays provide a way to examine the underlying
genetics of responders and nonresponders without any
of the assumptions or limitations used in candidate-gene
approaches. For most drugs with variable responses, little
is known about why they work in some patients and not
in others. Microarray analysis enables scientists to explore
the whole genome and to identify predictive markers of
disease and drug response. This may ultimately provide
more tailored, effective, and safer courses of treatment
and help avoid some of the over 100,000 annual fatalities
from adverse drug reactions in the U.S. alone.20
In a recent Phase III clinical trial by Novartis
Pharmaceuticals (Basel, Switzerland) expression profiles
were used to predict the success or failure of
Glivec®/Gleevec® treatment on chronic myelogenous
leukemia.21 Researchers analyzed gene expression
patterns from patients prior to treatment and
found a 31-gene “No Response” signature, which predicts
a 200-fold higher probability of failed therapy.
Similarly, in a Phase II clinical trial conducted at the
Dana Farber Cancer Research Institute (Boston, MA)
for the Millennium Pharmaceuticals (Cambridge,
MA) drug Velcade™ (generic name bortezomib),
researchers used GeneChip arrays to collect pharmacogenomic
data from myeloma patients treated with
the drug.22 Demonstrating the predictive power of gene
expression profiles, the scientists discovered a pattern
consisting of 30 genes that correlate with response or
lack of response to therapy. Clinical utility of biomarkers
will be further assessed in a Phase III trial.
As clinical researchers use genomic information and compare
their array data within and between laboratories or
hospitals, standardized array methodologies and data
reporting criteria will be essential. Affymetrix is actively
involved in a number of consortia aimed at providing
guidelines and standards for microarray applications. The
Microarray Gene Expression Data Society (MGED) has
taken the first step by developing data reporting guidelines,23,24 enabling scientists to properly compare data from
different experiments. However, guidelines will also need
to address variability in data generation and interpretation.
There are at least four key areas for microarray optimization
and standardization: study design, variation in platform,
analysis method variation, and “back-end” statistical
analyses.25 By standardizing each of these areas, microarray
analysis can be performed according to defined standards
and protocols necessary for regulated applications.
The way ahead
To improve human health, we need to shift the paradigm
from diagnosing and treating an existing disease to one in
which we predict disease susceptibility, determine individual
response to drugs, and focus on earlier detection, more
accurate diagnosis, and therapeutic management. This is
the very definition of personalized medicine, and it is where
GeneChip technology holds the greatest promise. While
grounded in basic laboratory research, advances in industrialization,
automation, and standardization have fundamentally
changed the use of GeneChip arrays, and have created
new applications for this highly flexible and scaleable technology.
By translating research findings into clinically relevant
information, GeneChip arrays are positioned to revolutionize
patient health care, as surely as Jacob and Monod’s
1961 discovery revolutionized modern biology.
References
-
Fodor SP, Read JL, Pirrung MC, Stryer L, Lu AT, Solas
D. Light-directed, spatially addressable parallel chemical
synthesis. Science 1991; 251:767–73.
- Fodor SP, Rava RP, Huang XC, Pease AC, Holmes CP,
Adams CL. Multiplexed biochemical assays with biological
chips. Nature 1993; 364:555–6.
- Pease AC, Solas D, Sullivan EJ, Cronin MT, Holmes CP,
Fodor SP. Light-generated oligonucleotide arrays for rapid DNA
sequence analysis. Proc Natl Acad Sci USA 1994; 91:5022–6.
- Armstrong SA, Staunton JE, Silverman LB, et al. MLL
translocations specify a distinct gene expression profile that
distinguishes a unique leukemia. Nat Genet 2002; 30:41–7.
- Ross ME, Zhou X, Song G, et al. Classification of pediatric
acute lymphoblastic leukemia by gene expression profiling.
Blood 2003; 102:2951–9.
- Kohlmann A, Schoch C, Schnittger S, et al. Pediatric
acute lymphoblastic leukemia (ALL) gene expression signatures
classify an independent cohort of adult ALL
patients. Leukemia 2004; 18:63–71.
- Steinman L, Zamvil S. Transcriptional analysis of targets
in multiple sclerosis. Nat Rev Immunol 2003; 3:483–92.
- Semizarov D, Frost L, Sarthy A, Kroeger P, Halbert DN,
Fesik SW. Specificity of short interfering RNA determined
through gene expression signatures. Proc Natl Acad Sci
USA 2003; 100:6347–52.
- Bleicher KH, Bohm HJ, Muller K, Alanine A. I. Hit and
lead generation: beyond high-throughput screening. Nat
Rev Drug Discov 2003; 2:369–78.
- Proudfoot JR. Drugs, leads, and drug-likeness: an analysis
of some recently launched drugs. Bioorg Med Chem
Lett 2002; 12:1647–50.
- Ramaswamy S, Golub TR. DNA microarrays in clinical
oncology. J Clin Oncol 2002; 20:1932–41.
- MacDonald TJ, Brown KM, LaFleur B, et al. Expression
profiling of medulloblastoma: PDGFRA and the
RAS/MAPK pathway as therapeutic targets for metastatic
disease. Nat Genet 2001; 29:143–52.
- Lapointe J, Li C, Higgins JP, et al. Gene expression profiling
identifies clinically relevant subtypes of prostate cancer.
Proc Natl Acad Sci USA 2004; 101:811–6.
- Huang E, Cheng SH, Dressman H, et al. Gene expression predictors
of breast cancer outcomes. Lancet 2003; 361:1590–6.
- West M, Blanchette C, Dressman H, et al. Predicting the
clinical status of human breast cancer by using gene expression
profiles. Proc Natl Acad Sci USA 2001; 98:11462–7.
- Beer DG, Kardia SL, Huang CC, et al. Gene-expression
profiles predict survival of patients with lung adenocarcinoma.
Nat Med 2002; 8:816–24.
- Notterman DA, Alon U, Sierk AJ, Levine AJ.
Transcriptional gene expression profiles of colorectal adenoma,
adenocarcinoma, and normal tissue examined by
oligonucleotide arrays. Cancer Res 2001; 61:3124–30.
- Twine NC, Stover JA, Marshall B, et al. Disease-associated
expression profiles in peripheral blood mononuclear
cells from patients with advanced renal cell carcinoma.
Cancer Res 2003; 63:6069–75.
- Shipp MA, Ross KN, Tamayo P, et al. Diffuse large B-cell
lymphoma outcome prediction by gene-expression profiling
and supervised machine learning. Nat Med 2002; 8:68–74.
- Lazarou J, Pomeranz BH, Corey PN. Incidence of
adverse drug reactions in hospitalized patients: a metaanalysis
of prospective studies. Jama 1998; 279:1200–5.
- McLean LA, Gathmann I, Capdeville R, Polymeropoulos
MH, Dressman M. Pharmacogenomic analysis of cytogenetic
response in chronic myeloid leukemia patients treated
with imatinib. Clin Cancer Res 2004; 10:155–65.
- Mulligan G, Kim S, Stec J, et al. American Society of
Hematology Annual Meeting, Philadelphia, PA, 2002.
- Spellman PT, Miller M, Stewart J, et al. Design and implementation
of microarray gene expression markup language
(MAGE-ML). Genome Biol 2002; 3:RESEARCH0046.
- Brazma A, Hingamp P, Quackenbush J, et al. Minimum information
about a microarray experiment (MIAME)—toward
standards for microarray data. Nat Genet 2001; 29:365–71.
- The Tumor Analysis Best Practices Working Group. Expression
profiling—best practices for data generation and interpretation in
clinical trials. Nat Rev Genet 2004; 5:229–37.
Mr. Dance is Senior Vice President, Product Marketing,
Affymetrix, 3380 Central Expy., Santa Clara, CA 95051,
U.S.A.; tel.: 408-731-5000; fax: 408-731-5441; e-mail:
[email protected].