The extensive workflow required to analyze DNA samples using next-generation sequencing (NGS) leaves many opportunities for the introduction of variability. To achieve accurate and precise results in an NGS assay, each stage of the protocol must be routinely optimized, validated and monitored. In this regard, well-characterized reference standards are an effective route to earlier detection of diseases, noninvasive monitoring and lower sample input, all of which push the technical limits of NGS.
Challenges of next-generation sequencing
NGS has become a universal tool in many industries, and most recently moved into the clinical arena for use in disease diagnosis. Laboratories need to optimize and validate their workflow in order to analyze patient-derived materials. They must demonstrate that instruments, apparatus, reagents and personnel comply with Clinical Laboratory Improvement Amendments (CLIA) guidelines and oversight by organizations such as the College of American Pathologists (CAP) and the American College of Medical Genetics and Genomics (ACMG) to ensure accuracy and consistency. As an example, the minimum data set requirements from the New York State guidelines are shown in Table 1.1
Table 1 – NGS minimum data set requirements from the New York State guidelines for somatic genetic variant detection
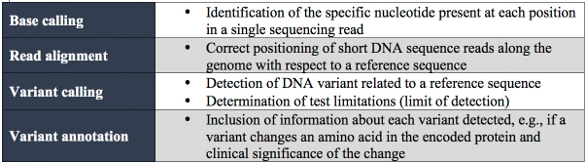
Meeting the evolving needs of NGS
Options that satisfy validation and optimization needs include cell lines, reference standards, patient samples and oligonucleotides. Testing methodologies are recommended based on a laboratory’s accreditation or requirements. New York State guidelines state that researchers must “Establish the analytical sensitivity of the assay for each type of variant detected by the assay. This can initially be established with defined mixtures of cell line DNAs (not plasmids), but needs to be verified with 3–5 patient samples.”1
The NGS workflow challenges are:
- Tumor sample—heterogeneity (stromal contamination), low quantity and poor sample quality are important factors that impact the final assay results
- DNA extraction—extraction from low-quality and low-quantity samples and accurate assessment of quantity are challenges presented by patient-derived samples
- Library preparation—specific library preparation approaches are tailored to the goals of the experiment and the quantity and quality of the sample
- Sequencing—read length and type (paired-end vs single-end) and sample multiplexing are determined by the library fragment size and the coverage required for detection
- Bioinformatics—on-sequencer analysis may be employed, or data may be exported into either commercially available software or a laboratory-built pipeline
- Analysis and interpretation—after generating a list of variant calls and their corresponding frequencies, database annotations, statistics and metadata may be incorporated to improve understanding and interpretation of assay results.
Preanalytical variability
Tumor sample and DNA extraction
High-quality genetic material is needed to generate interpretable data. Sample age, method of preservation and storage conditions all impact quality. Suitable extraction kits and methods are determined by sample quality. For example, consider formalin-fixed, paraffin-embedded (FFPE) tissue samples. These samples must be extracted using a technique that first removes the paraffin via chemical dissolution and that can accommodate degraded genomic material. When using diagnostic-grade kits such as the QIAamp DSP DNA FFPE tissue kit (QIAGEN, Valencia, Calif.), certain parameters may need to be optimized before an approved protocol can be established. During optimization, a well-characterized standard is used to evaluate how protocol modifications affect the resulting DNA yield. One such product, FFPE reference standards from Horizon Discovery (Cambridge, U.K.), are prepared using highly homogeneous cell densities that yield a consistent and reproducible quantity of DNA. (Note: Horizon Discovery reference standards are approved for research use only.) Data from Horizon shows that when DNA is extracted from these standards using five different commercially available protocols, significant variability in yield is observed, as shown in Figure 1. Use of a cell-line-derived, renewable reference standard helps ensure that patient samples are not compromised.
Figure 1 – DNA recovery from total theoretical yield from FFPE reference standards.Following extraction, accurate DNA yield must be determined in order to input the appropriate amount of material for downstream sequencing library preparation, as well as to understand theoretical allele frequency thresholds (see below). While spectroscopic methods like UV/VIS provide adequate estimation at concentrations higher than 10 ng/μL, fluorometric quantification will always be more accurate and specific (measuring DNA only).
Analytical variability
Sequencing
MiSeq (Illumina, San Diego, Calif.) and Ion Personal Genome Medicine (PGM) (Ion Torrent/Life Technologies, Carlsbad, Calif.) benchtop sequencers are often used for the analysis of patient-derived samples.2 Optimal sequencing depth, or coverage, is critical: if there is not sufficient coverage of the position(s) of interest during sequencing, it may be difficult or impossible to interpret the results.
The Quantitative Multiplex reference standard was sequenced using the AmpliSeq Cancer Hotspot Panel V2 (Life Technologies, Carlsbad, Calif.) by three different NGS laboratories (see Table 2). For the 11 engineered, validated mutations present in the sample, those below 5% were routinely not detected. Even in the laboratory of Partner C, where the highest average coverage was achieved, the 5% KRAS G12D mutation was not detected. A second NGS platform and/or entire workflow is commonly used to validate detected mutations and their allelic frequencies. This helps to account for inherent biases associated with each platform (i.e., homopolymer errors), and allows confirmation of variants outside of the limit of detection of Sanger (approximately 20%).3
Table 2 – NGS sequencing results*
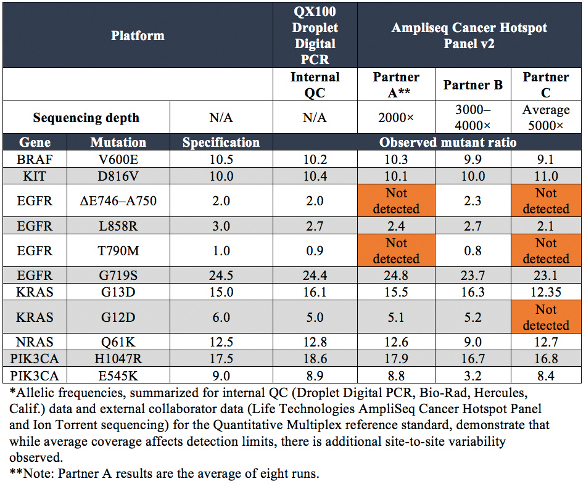
Bioinformatics
The databases, algorithms and operators employed during informatics can have a significant influence on the resulting data. This is demonstrated by results compiled by researchers from the Genome in a Bottle Consortium, a public/private/academic collaboration hosted by the National Institute of Standards and Technologies (NIST), which aims to standardize and improve human whole genome sequencing (see Figure 2).4
Figure 2 – One data set with five bioinformatic workflows. GATK, Genome Analysis Toolkit; SOAPsnp, part of Short Oligonucleotide Analysis Package; SNVer (full name); SAMTools, Sequence Alignment/Map Tools; GNUMAP, Genomic Next-generation Universal MAPper.Data was analyzed from 15 exomes, with five alignment and variant-calling pipelines, and significant differences were observed: less than 60% of calls were shared by all pipelines.4 As seen for the molecular side of the assay, a well-characterized reference standard with known allelic frequencies allows clinicians to ensure that the informatics are not introducing unwanted bias, and that changes made to the pipeline over time (e.g., as software is upgraded) do not affect downstream results.
Once generated, short sequencing reads are first evaluated for quality (using a Q score, similar to a Phred score) before being aligned to a human reference genome. Alignment of these short reads to a reference sequence allows for the identification of single-nucleotide polymorphisms (SNPs) and insertions/deletions (INDELs). In brief, a common bioinformatics pathway includes: 1) quality control (e.g., FastQC, a software application from the Babraham Institute in Cambridge, U.K.); 2) de-duplication (removing PCR-duplicated molecules) and trimming, if needed; 3) alignment and 4) variant calling.
On-machine software for the MiSeq and Ion PGM sequencers provides a straightforward means of generating variant call data. Depending on the goals or flexibility of the assay, an external, off-the-shelf software package or custom-developed pipeline is also an option. There may be variations among the analysis software options for parameters like alignment stringency (i.e., how closely the sequence must match the reference) or threshold of coverage required to make a variant call (e.g., position must be sequenced at coverage of 4× or greater). For these parameters, it is important to evaluate how changes to the pipeline affect the final data output. A predictable, orthogonally validated reference standard makes this easy to achieve for software updates.
Calculating theoretical limit of detection
To enable disease discovery, it is imperative that NGS assays are validated for the detection of the lowest possible allelic frequencies. This is particularly true concerning the analysis of cell-free DNA (cfDNA) for oncology-relevant mutations. In these patient samples, copies of the disease-relevant mutant DNA may be very low relative to the healthy/wild-type DNA. This is also the case with the early detection of organ rejection following transplantation, noninvasive prenatal analysis, and with disease monitoring during treatment. Reference standards are perhaps even more useful for addressing the challenge faced by those adopting NGS for such clinically relevant analyses. Specifically, as alluded to earlier, the theoretical limit of detection for an NGS experiment is influenced by such parameters as molecular uniqueness (deduplication), input amount and coverage (see Figure 3 and equations below).5
Figure 3 – Parameters that affect theoretical limit of detection of an NGS experiment.Molecular uniqueness
In a traditional amplicon panel, short amplicons are generated with the same forward and reverse primer sequences, targeting a specific region of interest (gray “reads” represented in Figure 3). The identical ends of these sequences make it difficult to disambiguate unique molecules from those “duplicated” during PCR amplification. Ideally, only unique molecules would be used to calculate allelic frequencies of mutations in order to avoid errors and bias from amplified DNA. Capture-based libraries (navy blue “reads” in Figure 3) overcome this issue because DNA is first randomly sheared, generating random start/stop sites that can be disambiguated or de-duplicated. However, the input amount required for capture-based libraries is generally higher.
Importantly, because coverage distribution is not uniform across a sample, it may be necessary to sequence much deeper to achieve the desired coverage at each position of interest. The calculations shown in the equations below demonstrate the theoretical limit. When experimental noise is factored in, the actual limit of detection may be much higher. Determination of an assay’s limit of detection using a known reference standard is good practice and is required by CLIA to process patient samples.
Input amount
The amount of input material in an NGS experiment greatly influences the lowest possible allelic frequencies that may be detected. Essentially, it is not possible to cover the position of interest more times than there are copies of DNA in a biological sample. It is not unusual for an informatics pipeline to remove PCR duplicates (as described above) and require a minimum of four reads to show the mutation of interest in order to make a variant call. Therefore, if the number of copies of DNA present in a sample is estimated using 3.3 pg for a single, haploid copy, then for DNA samples of 10 ng, the theoretical limit of allelic frequency detection is near 0.1%:

As the input amount increases, the theoretical detection limit decreases. This is a theoretical calculation; when experimental noise is taken into account, the actual limit of detection may be much higher.
Coverage
Sequencing coverage also plays a role in an assay’s limit of detection. If one is not limited by input material, then the denominator of the equation below is not restricted by molecules but, rather, becomes reads exclusively:

Again, assuming four reads that contain the mutation of interest are required to detect allelic frequencies of 0.01% or lower, this positon must be sequenced at least 40,000×. Importantly, since coverage distribution is not uniform across a sample, it may be necessary to sequence significantly deeper to achieve coverage of 40,000× at each position of interest.
Implementation in the laboratory
As NGS continues to be pushed to its theoretical limits, it is necessary to evaluate protocol performance with known standards before implementation into the clinical workflow. Optimizing assay parameters is perhaps most critical when the outcome can affect the downstream stratification, diagnosis or treatment of patients. Reference standards can potentially play a key role in engineering checkpoints for validation into an NGS workflow, from preanalytical steps through informatics analysis. Routine validation ensures consistency between reagent lots and personnel and, when a reference standard is used across multiple laboratories and workflows, the data may be shared and used to improve platforms, kits, protocols and assay development. Orthogonally validated, well-characterized human, genomic DNA reference standards offer a renewable resource with which this can be achieved.
References
- New York State Department of Health. Updated and revised: Oncology—molecular and cellular tumor markers. “Next generation” sequencing (NGS) guidelines for somatic genetic variant detection; http://www.wadsworth.org/labcert/TestApproval/forms/NextGenSeq_ONCO_Guidelines.pdf. Accessed Jun 22, 2015, published Mar 2015.
- Meldrum, C.; Doyle, M.A. et al. Next-generation sequencing for cancer diagnostics: a practical perspective. Clin. Biochem. Rev. 2011, 32(4), 177–95.
- Quail, M.A.; Smith, M. et al. A tale of three next generation sequencing platforms: comparison of Ion Torrent, Pacific Biosciences and Illumina MiSeq sequencers. BMC Genomics 2012,13, 341.
- O’Rawe, J.; Jiang, T. et al. Low concordance of multiple variant-calling pipelines: practical implications for exome and genome sequencing. Genome Med. 2013, 5(3), 28.
- Armstrong, J.A. Heterogenous DNA sequencing and the lower limits of minor allele frequency sensitivity; https://cofactorgenomics.com/heterogenousdna-sequencing-lower-limits-minor-allelefrequency-sensitivity/. Accessed May 4, 2015, published Oct 2014.
Natalie A. LaFranzo, Ph.D., is product manager— next-generation sequencing scientist, Horizon Discovery Ltd., 7100 Cambridge Research Park, Waterbeach, Cambridge, U.K.; tel.: +44 (0) 1223 655 580; e-mail: [email protected]; www.horizondiscovery.com. Horizon’s reference standards are for research use only and not for diagnostic procedures.