Commercial LIMS have been available for more than 20 years and have become key elements in the laboratory informatics framework. During these years there have been many changes within the LIMS industry, many of them driven by rapidly developing technologies. At the same time, evolving business requirements and pressures have been crucial factors in making LIMS the sophisticated business-critical systems they are today.
Bringing state-of-the-art technologies into the laboratory
The major technological developments in the LIMS arena have included a shift from mainframe or mini systems (i.e., the DEC VAX hardware series) to PC and server-based solutions. This has been accompanied by a change in operating systems, and the move from proprietary databases to standardized database management systems such as Oracle (Oracle Corp., Redwood Shores, CA) and SQL Server (Microsoft Corp., Redmond, WA).
Quite possibly, however, the technological changes that have had the biggest impact on the LIMS industry are those related to networking. We have moved from standalone machines to local area networks (LAN) and wide area networks (WAN), and from there to the adoption of the Internet and virtual private networks (VPN). Clearly, this has had a tremendous impact on our ability to share systems and IT resources across geographically dispersed facilities.
Responding to evolving business requirements
At the same time, the LIMS industry has been affected just as strongly by constantly evolving business requirements. One of the key business pressures over the past 25 years has been an increase in globalization: of markets, of production, and of R&D. For LIMS users, this has meant that laboratories in the manufacturing or process industries are working on products that will be sold to multiple markets and which may therefore be subject to multiple quality specifications. On the production side, it means that quality managers need to manage information generated by manufacturing plants that might be on opposite sides of the globe. For research and development, the challenge is to ensure that geographically dispersed research teams can effectively exchange and manage research data. Organizations are also adapting to stiffer competition, often by increasing manufacturing efficiency and reducing the time-to-market of new products. Effective management of laboratory data and information is essential in meeting these challenges.
In addition, the laboratory industry has dynamically adapted to evolving government policies and regulatory requirements—often caused by concerns over human health, safety, and welfare. The seemingly ever-changing fortunes of the environmental sector illustrate this perfectly.
Managing exponentially growing volumes of data
Finally, laboratories are confronted by the exponential growth in data generation rates. Not only do these data and information need to be stored and retrieved, but organizations need to maximize the value of the data and information at their disposal.
LIMS in the modern laboratory: benefits and limitations
All of these pressures have led to the development of the modern LIMS: sophisticated, business-critical IT systems whose function extends well beyond the simple management of sample, test, and result data, and which have helped laboratories and businesses to address their ever-changing information and quality management issues. Nevertheless, despite the crucial role that LIMS play in the modern laboratory, information management in analytic environments is far from perfect. Silos of information still exist within laboratory organizations, access to data and information can still be a problem, and there are still significant numbers of laboratories running without LIMS. So why is this the case? Why have LIMS not become the ubiquitous solution to all laboratory informatics needs, and why do organizations still have issues with data and information management?
One reason could be that along with the development of LIMS there has been an equivalent development of other laboratory informatics systems. For example, instrument data systems have become increasingly sophisticated to the point where users of these systems can legitimately question the need for LIMS. There has also been the development of alternative laboratory informatics systems such as electronic laboratory notebooks (ELN) and scientific data management systems (SDMS); even corporate or enterprise systems such as enterprise resource management systems have pushed into the laboratory informatics space.
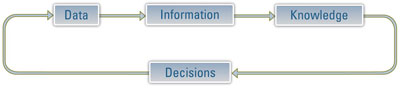
Figure 1 - For laboratories and knowledge-based organizations, the knowledge-management cycle is of crucial importance. An analytical environment should efficiently use data to create information, information to generate knowledge, knowledge to support decisions, and decisions to shape the type of data generated.
Altogether, it is safe to say that today’s laboratory manager has a wide choice of laboratory informatics solutions and approaches, and this certainly sounds like a fortuitous situation. Yet, despite this mulititude of offerings, access to data and information is not as seamless as it should be. The success of laboratories and lab-centric organizations rests largely on their ability to manage knowledge creation and use. Ideally, data are used to create information, information generates knowledge, knowledge is used to make decisions, and those decisions affect the data to be generated (see Figure 1). Yet in many laboratories and enterprises, this crucial cycle is not working effectively.
Laboratory informatics: models and visions
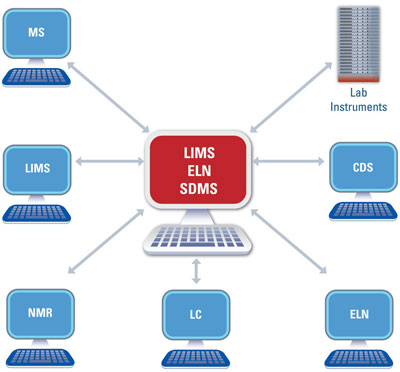
Figure 2 - Laboratory informatics is usually modeled as a single central system, which functions as a hub for multiple other systems.
When faced with this dilemma, it is commonplace to suggest that if the knowledge-generation cycle is working imperfectly, this must be due to flawed business processes and/or inappropriate IT systems. These are important factors, which have been discussed at length in this and other publications. However, there is another set of reasons why the data/information/knowledge/decision process may be impaired. These explanations concern the lack of understanding of laboratory informatics strategy and oversimplification of the laboratory informatics model. Laboratory informatics is usually modeled as a single central system, which functions as a hub for multiple other systems (see Figure 2). Such a system-centric architecture may be a vast oversimplification, which erroneously gives one element more importance than any others.
The case for a multilayer approach to laboratory informatics
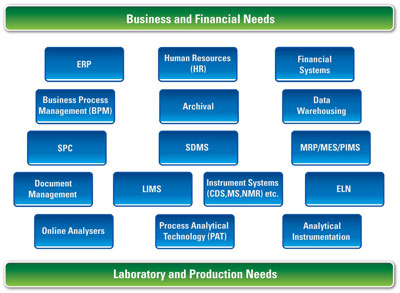
Figure 3 - A typical QA/QC laboratory, like other types of laboratories, has a complex information architecture, which is best described using a multilayer model. Such models do not revolve around a specific IT system, but around the laboratory’s identified business needs.
In reality, laboratory informatics has a far more complex architecture, which is more accurately defined by using multilayer models (see Figure 3). The key to these models—and their key difference from system-centric models—is that they revolve around the laboratory’s identified business needs and the systems used to help meet those needs. Such needs may be financial, analytical, production, research, quality, etc. What distinguishes these models is that they are not hierarchical; there is no assumption that one system is more important or dominant than others. By enabling us to focus on the true informatics needs of a laboratory, they often expose that what were previously considered mutually exclusive systems actually coexist and have a role to play within the overall informatics architecture and strategy. For example, it is quite possible that LIMS, ELN, and SDMS may all be necessary at the same time, and the multilayer approach can be used to illustrate their respective roles within the same laboratory.
Understanding the needs of a specific laboratory
Once the laboratory starts to look at its informatics architecture in this way, it becomes clear why no single system can meet all of their informatics requirements. It becomes easier to gauge the role and importance of each type of system, and how it contributes to the overall informatics architecture. In addition, this model helps define a laboratory’s precise integration needs, beyond the simple huband-spoke integration implied by the system-centric architecture.
At the same time, using the multilayer model puts the laboratory in a position to establish a complete informatics strategy based on a clear view of what needs to be achieved and how it can be achieved. This must be combined with an understanding of the relative strengths of the various systems that may be required. For example, ELN are typically thought of as good for experimental design, handling unstructured data and information and managing research collaboration, while SDMS are most suitable for the consolidation of data, information, knowledge management, and knowledge asset realization. LIMS are powerful platforms to manage structured data and information; sample tracking and management; and workload, resource, and asset management.
Putting the model into practice
This model can serve as a basis to create an overall laboratory informatics architecture that meets the needs of laboratories and organizations, as can be seen by looking at the role of LIMS with the research environment. The summary of the strengths of the various systems outlined above would seem to reinforce the generally held view that LIMS work well within the QA/QC or routine testing environment, while ELN are more suited to the research environment. However, exceptions abound. For instance, in research environments where high-throughput screening, compound libraries, genetic screening, or bio-banking are used, it is natural to see an increasing emphasis on chain of custody, usage profile of specimens, source, and storage location—and a concomitant emphasis on sample management and LIMS.
As an example, looking in more detail at the system architecture required for genetic research, it becomes apparent that there may be automated sample storage systems, liquid handling and plate handling systems, sequencing and sequencing analysis systems; in addition to LIMS to handle sample management, and possibly SDMS to act as a knowledge collection and management resource. None of these systems is more important than the others, nor is it absolutely necessary to integrate one system with all of the others. What is necessary is precisely defining the goals of the laboratory and carefully evaluating the strengths and limitations of each type of system. Such a comprehensive, multilayer approach enables managers to define and implement a laboratory informatics infrastructure capable of meeting the defined needs of the organization.
Dr. Wood is Executive Director of Marketing and Education, STARLIMS Corp., 4000 Hollywood Blvd. S515S, Hollywood, FL 33021, U.S.A.; tel.: 954-964-8663; fax: 954-964-8113; e-mail: [email protected]; home page: www.starlims.com.