At the heart of every scientific research and development effort is a significant amount of data. Whether in the form of chemical structures or biological sequences, images, or text, data increase knowledge; support decision-making; and drive the discoveries that lead to new drugs, consumer products, and industrial materials. While advances ranging from high-throughput experimentation (HTE) and in vivo imaging to next-generation sequencing mean that R&D enterprises generate more detailed data than ever, the volume that now needs to be managed is overwhelming. There is too much to analyze, standardization is lacking, and diverse and disparate data sources make it difficult for researchers to integrate and share their findings.
Although it sounds like an information technology (IT) problem, it is not that simple. While IT needs to play a central role in enabling better management and integration of scientific data, domain-savvy end users—including chemists, biologists, formulators, materials scientists, informaticians, and other key stakeholders in the R&D process—must also be given enough flexibility to manipulate and share information in a form most suited to their unique research methods.
Traditional solutions
Two key challenges have to be addressed by R&D organizations. First, to improve productivity and speed innovation, researchers and product developers must spend more time doing high-value work and less time searching for data, preparing information for analysis, manually creating reports, and bouncing back and forth between multiple applications and systems. These time-consuming and mundane tasks can and should be automated. Second, organizations need to find better ways to integrate, access, and understand the scientific data being generated, allowing stakeholders throughout the enterprise to make smarter decisions and speed time-to-innovation.
Many existing technologies, such as business intelligence (BI) and work flow automation tools, streamline operational processes and make corporate data more accessible and understandable for end users. The critical issue is that scientific data are completely different than standard corporate data. For one, scientific data are highly complex and far more diverse in terms of the types and formats of data that come into play.
For example, traditional BI typically deals with a well-defined warehouse structure involving rows and columns of data. This is a fairly static metadata model with orderly hierarchies and relationships that are easy to understand, process, and query. In contrast, scientific data include both structured and unstructured content ranging from standard row- and column-based data sets to scientifically meaningful text, detailed images, and two- and three-dimensional molecular structures. Scientific data may also be generated by a host of applications and devices, ranging from sophisticated software systems to laboratory equipment such as microscopes and analytical instruments. Furthermore, scientists do not ask the same questions every day. Creating and testing new hypotheses is not like viewing a weekly sales report. Queries of scientific data are ad hoc by nature, necessitating very nimble and flexible analytic capabilities.
The same goes for process automation. The best practices of a scientific organization must be captured and reused. Because scientific and product development processes are constantly evolving, however, organizations need to be able to easily reorder or remix process components as the needs of individual researchers or the project change.
In essence, true scientific business intelligence requires a solution that can bridge the gap between scientific research and IT—one that combines cutting-edge information management with capabilities that support the way scientists and other research project participants actually work.
Enterprise platform for scientific informatics
The data management requirements of the modern R&D enterprise are causing organizations to move beyond application and discipline-centric informatics solutions that trap information in silos, create barriers to collaboration, and add unnecessary effort and expense to the discovery process. The Pipeline Pilot® platform for scientific informatics (Accelrys, San Diego, CA) was designed with this broader scope in mind. Built on a services-based, open architecture, the platform supports the plug-and-play integration of diverse systems, applications, and data sources, coupled with the ability to manipulate and analyze complex scientific information. This end-to-end, enterprise-level approach:
- Enables scientists and other project stakeholders to more easily access, analyze, report, and share data across departments and disciplines
- Releases IT from the burden of manually supporting the varied requirements of multiple information consumers
- Allows the organization as a whole to extract maximum value from the many sources of scientific knowledge available to it, both internal (i.e., data generated through experiments, modeling, and simulation, etc.) and external (from publicly available databases or published literature).
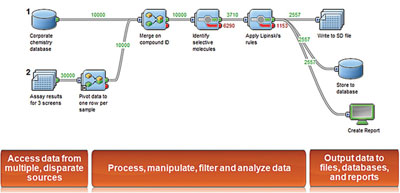
Figure 1 - Pipeline Pilot protocol.
Pipeline Pilot’s 20+ component collections serve as the scientific building blocks of the informatics platform. These component collections permit both generic and science-specific data processing spanning biology, chemistry, materials science, modeling, and simulation. The components that comprise each collection are central to the principal capability of Pipeline Pilot: data pipelining (see Figure 1). Essentially, data pipelining enables users to link components together in a simple drag-and-drop environment, integrating diverse data sets and defining data processing protocols to automate complex scientific work flows. This automation technology is simple enough to use that scientists and other project stakeholders can easily create and customize their own work flows without requiring an IT programmer to help. Because it is modular in nature, work flow steps can be reused and rearranged to fit the needs of particular researchers or projects, allowing for the process flexibility so critical to R&D activities. Finally, because data from legacy systems, third-party applications, and even external data sources can be integrated via Pipeline Pilot, there is no need for researchers to become experts of multiple software tools to do their jobs; they can access everything they need from a central system.
This is the essence of scientific business intelligence—information management capabilities that address the data integration and collaborative requirements of large, global enterprises, and the scientific breadth and depth that empower individual researchers across the organization to work more efficiently without compromising their ability to innovate.